MVG-Splatting: Multi-View Guided Gaussian Splatting with Adaptive Quantile-Based Geometric Consistency Densification
MVG-Splatting can achieve more fidelity rendering results.
MVG-Splatting can achieve more fidelity rendering results.
Abstract
In the rapidly evolving field of 3D reconstruction, 3D Gaussian Splatting (3DGS) and 2D Gaussian Splatting (2DGS) represent significant advancements. While 2DGS compressed 3D Gaussian primitives into 2D Gaussian surfels effectively enhances the quality of mesh extraction, it can lead to a decrease in rendering quality. Additionally, unreliable densification processes and the calculation of depth through the accumulation of opacity can compromise the detail of mesh extraction. To address this issue, we introduce MVG-Splatting, a solution guided by Multi-View considerations. Specifically, we integrate an optimized method for calculating normals, which, combined with image gradients, helps rectify inconsistencies in the original depth computations. Additionally, utilizing projection strategies akin to those in Multi-View Stereo (MVS), we propose an adaptive quantile-based method that dynamically determines the level of additional densification guided by depth maps, from coarse to fine detail. Experimental evidence demonstrates that our method not only resolves the issues of rendering quality degradation caused by depth discrepancies but also facilitates direct mesh extraction from dense Gaussian point clouds using the Marching Cubes algorithm. This approach significantly enhances the overall fidelity and accuracy of the 3D reconstruction process, ensuring that both the geometric details and visual quality.
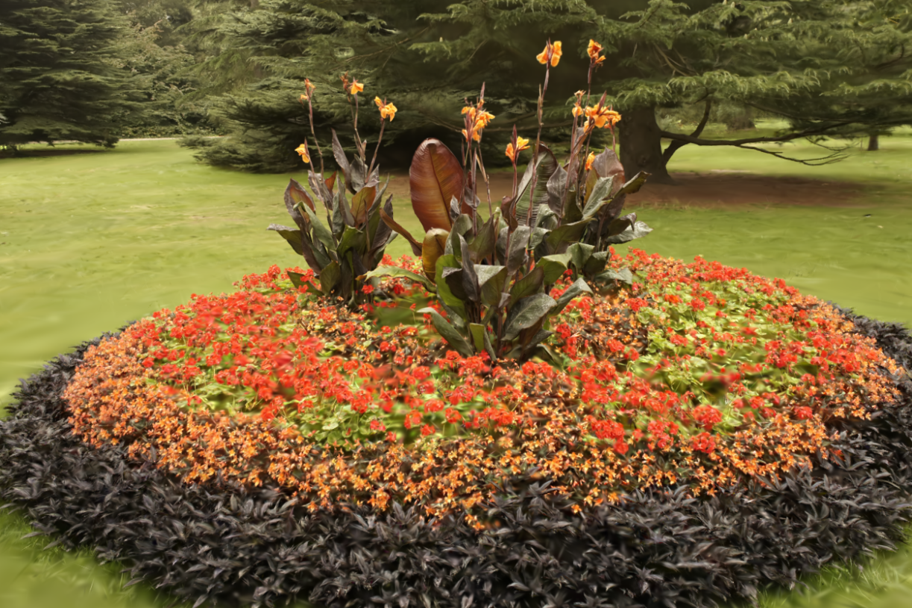
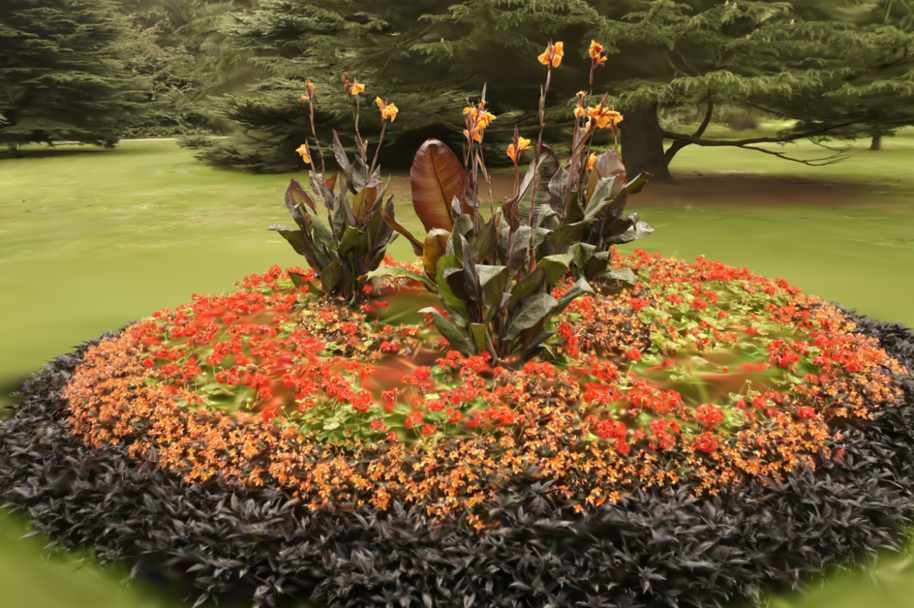
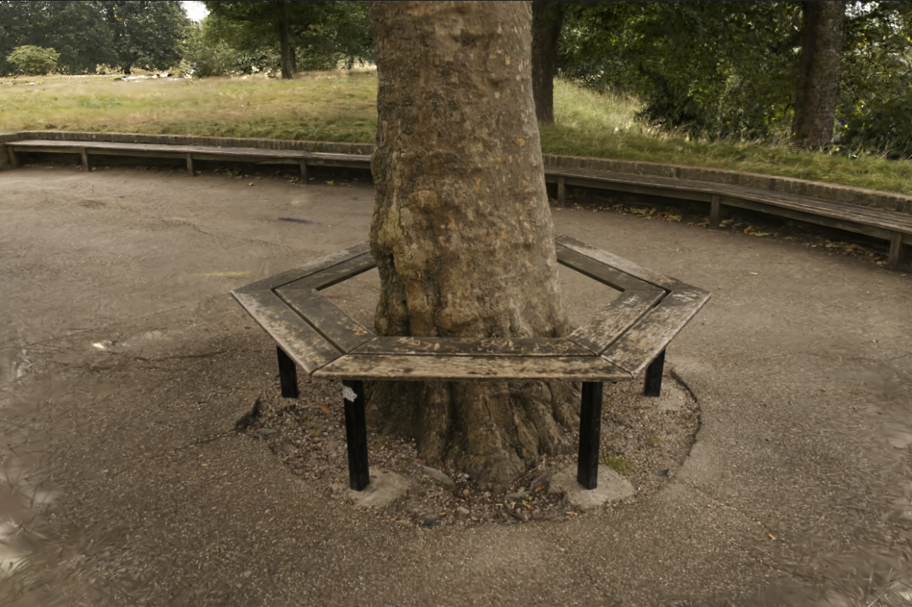
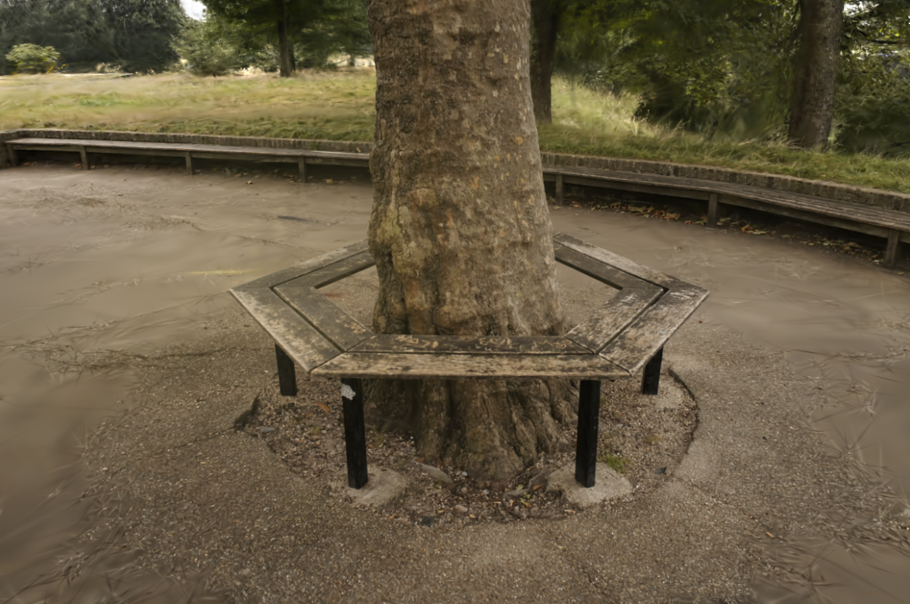
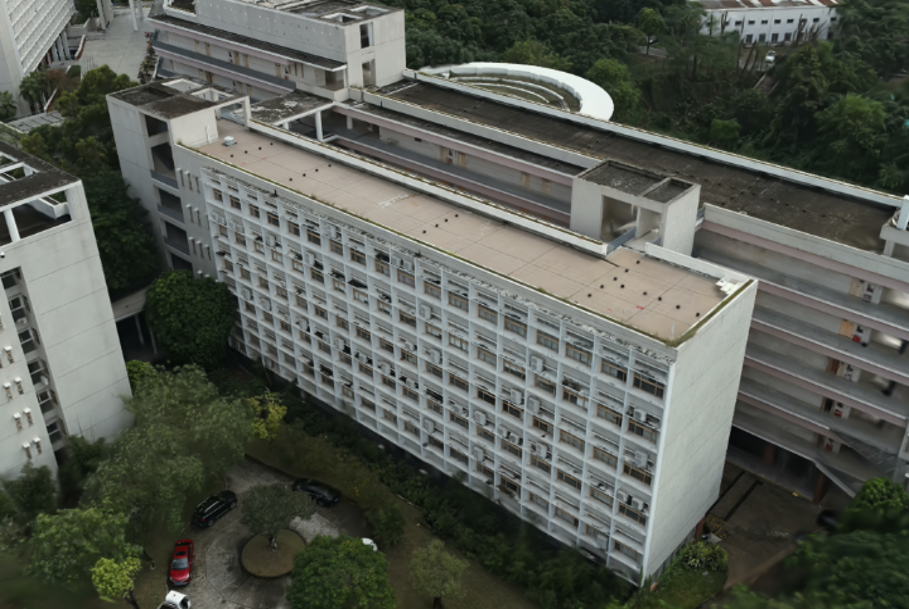
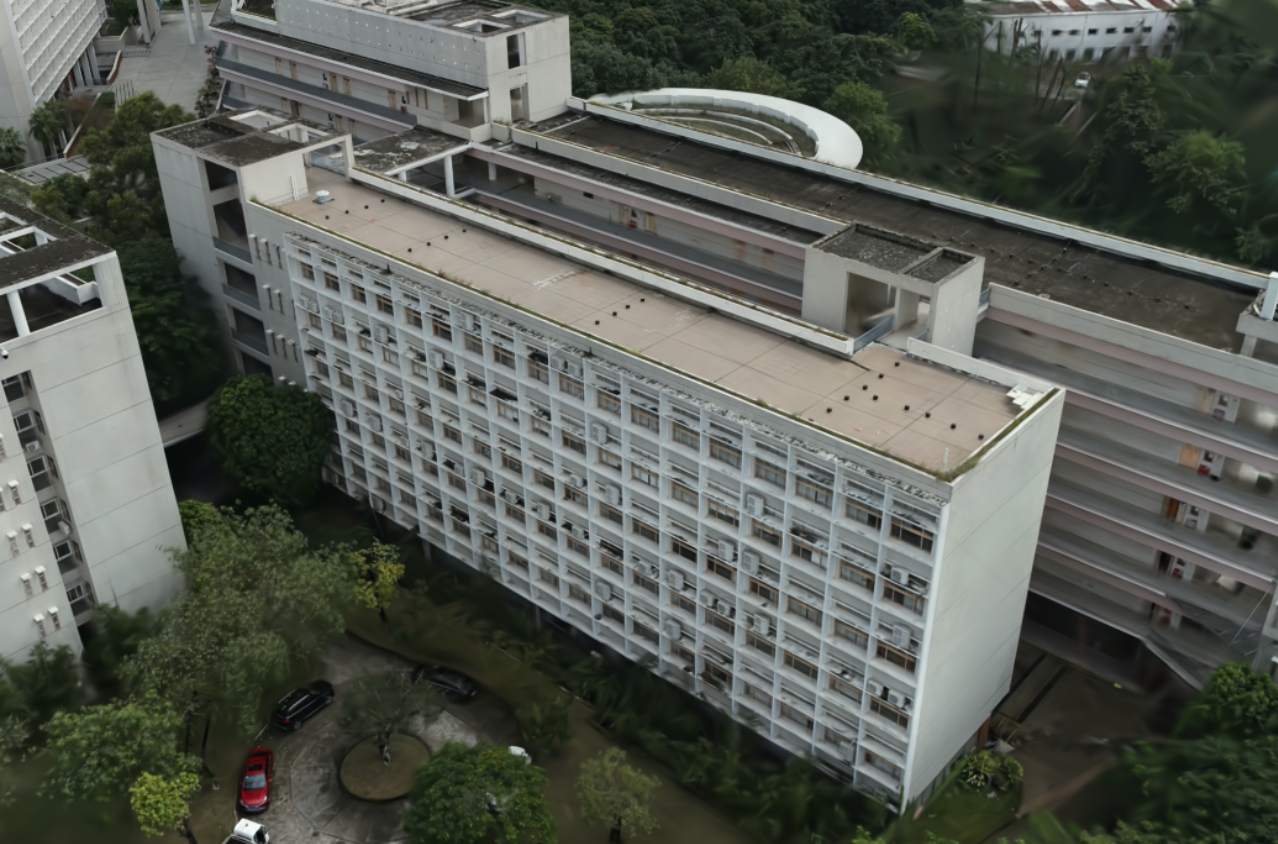
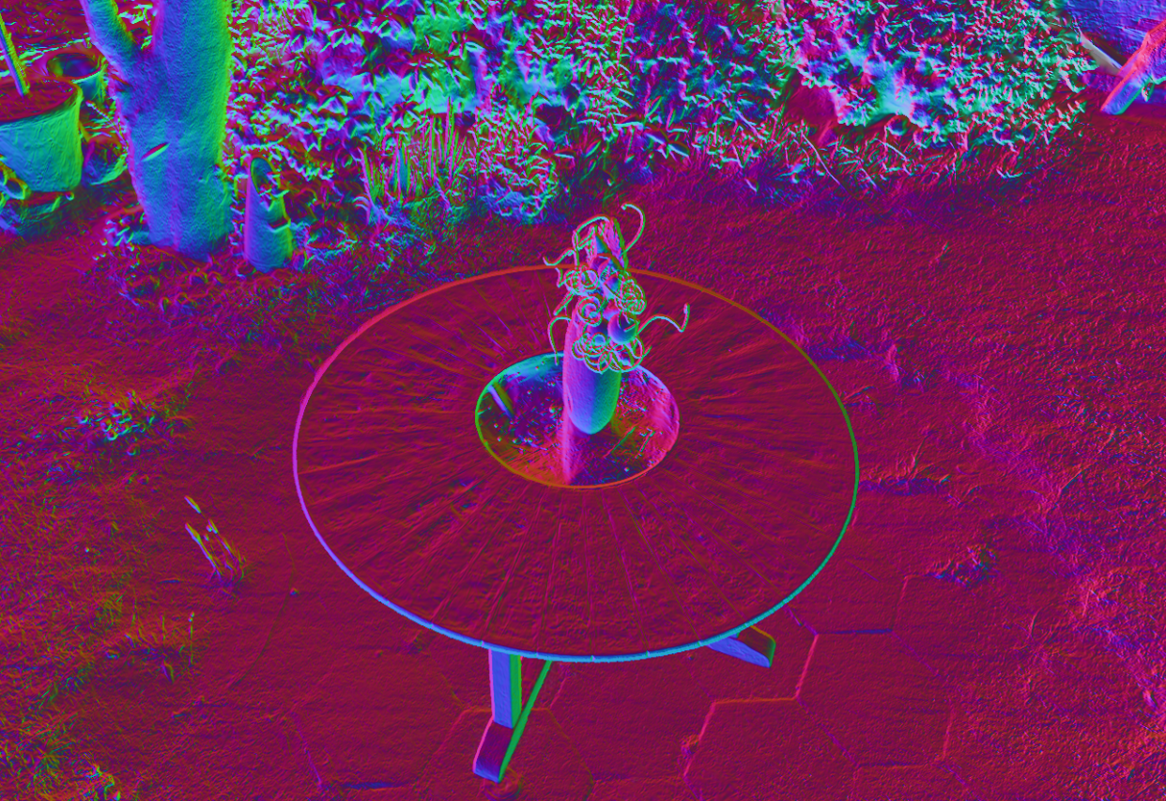
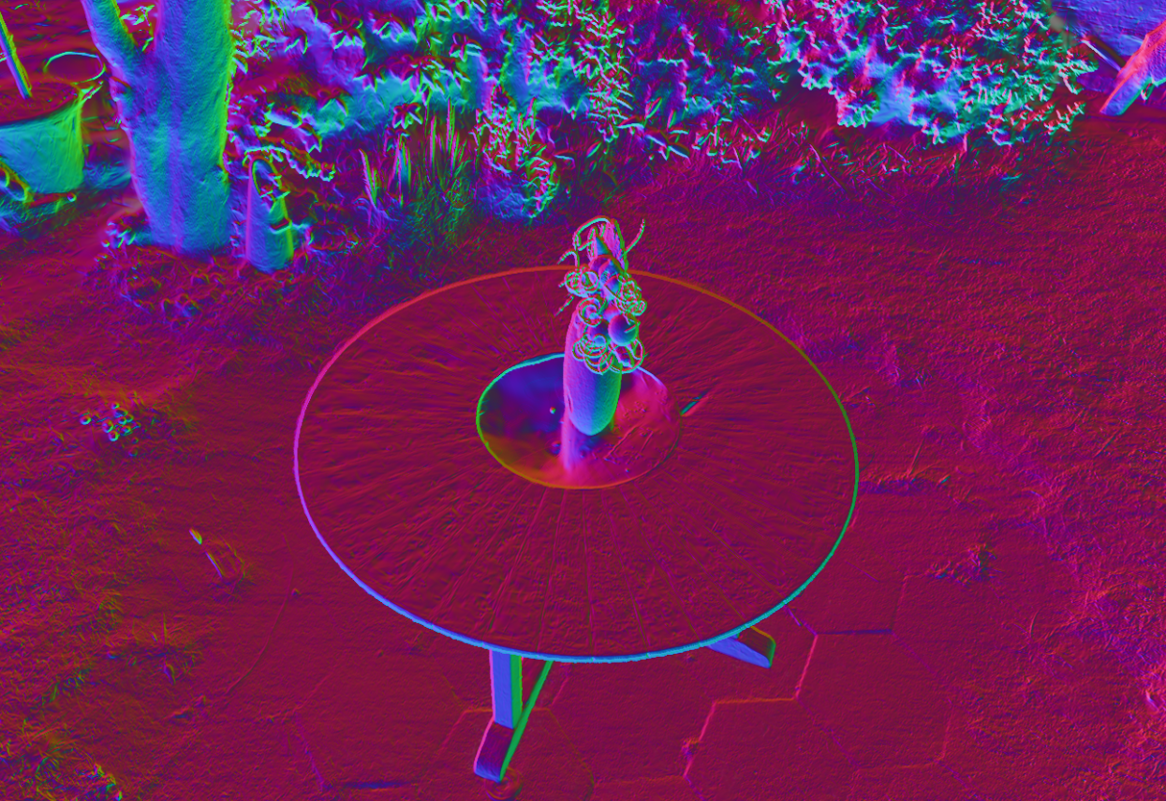
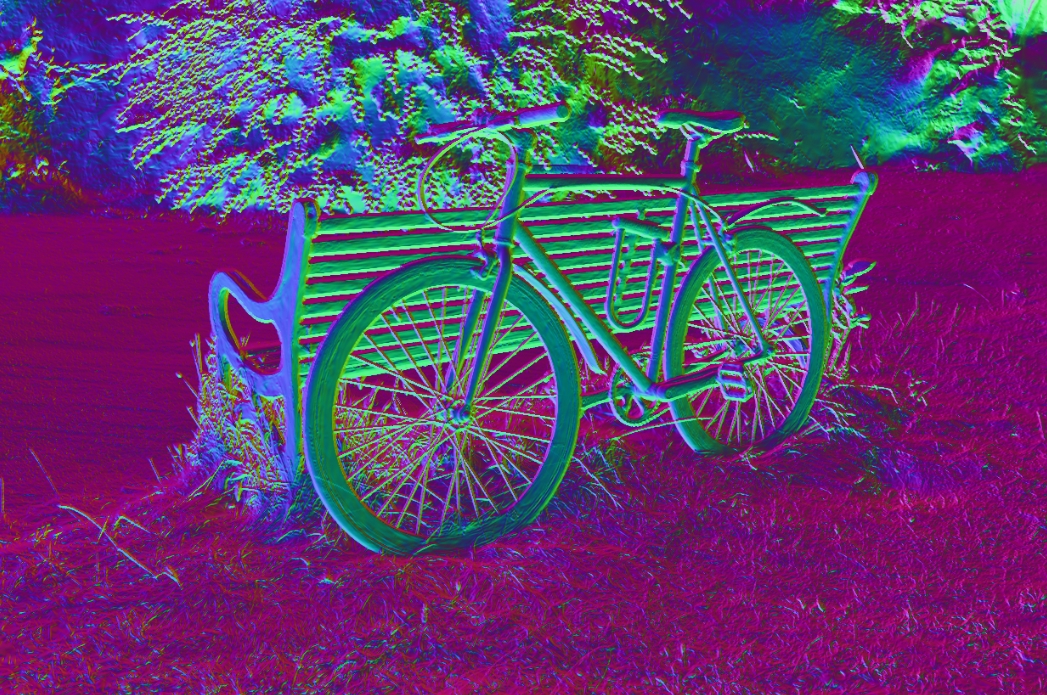
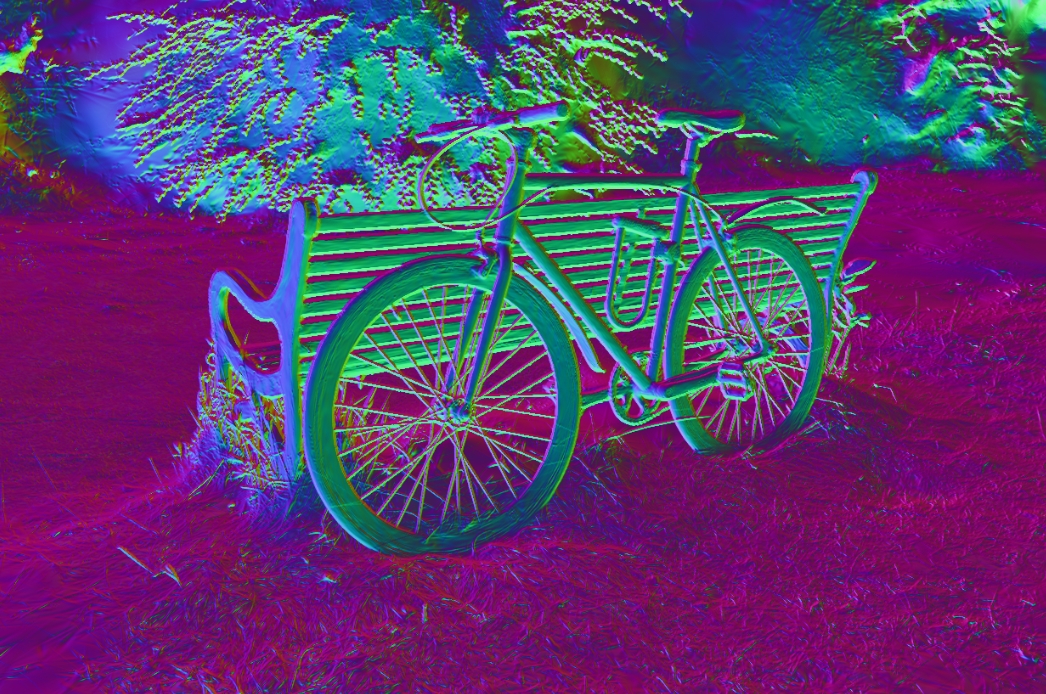
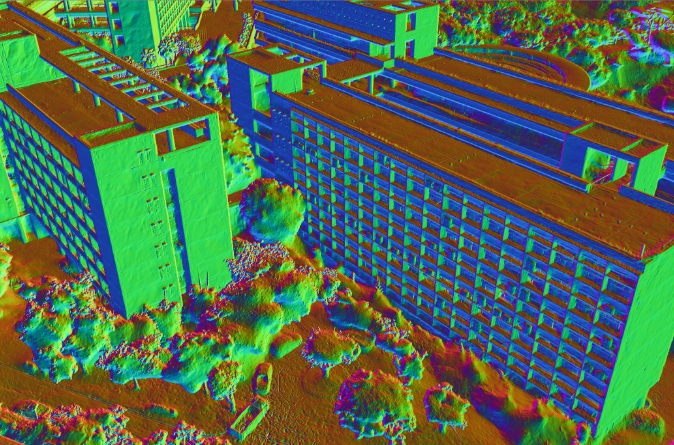
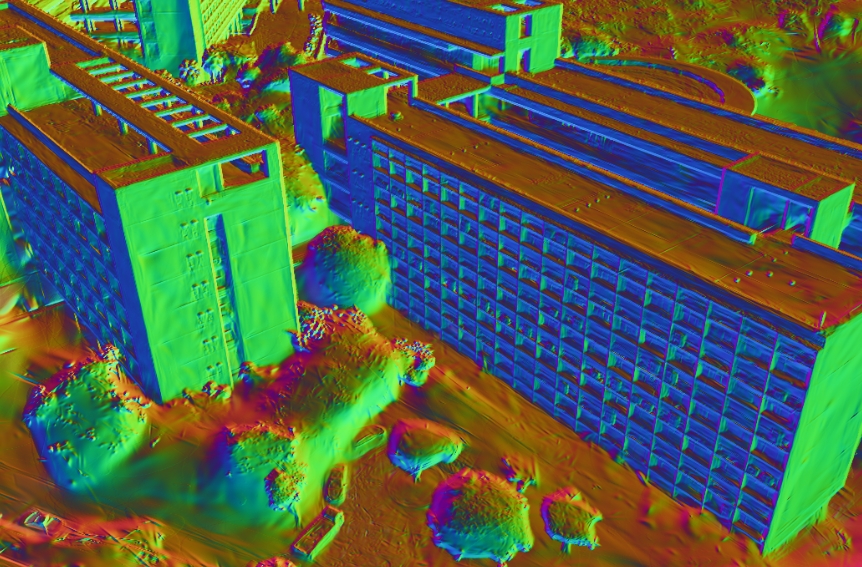
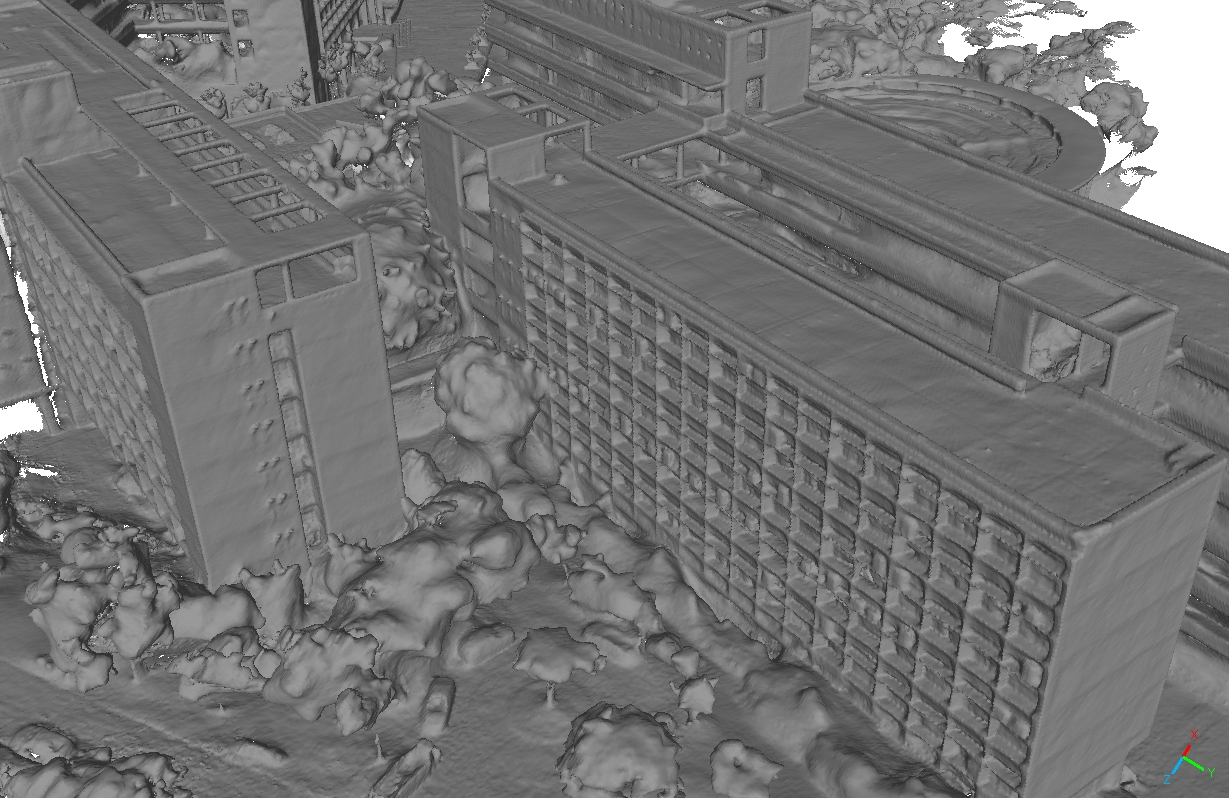
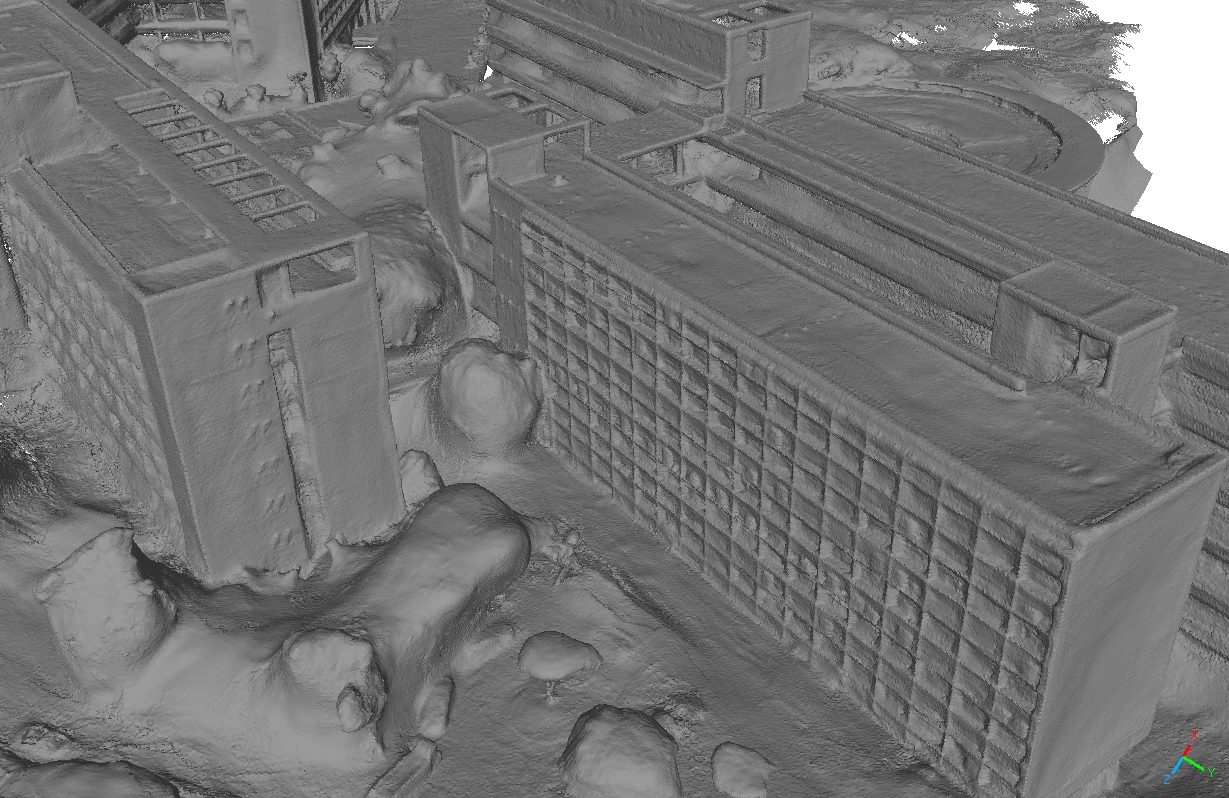
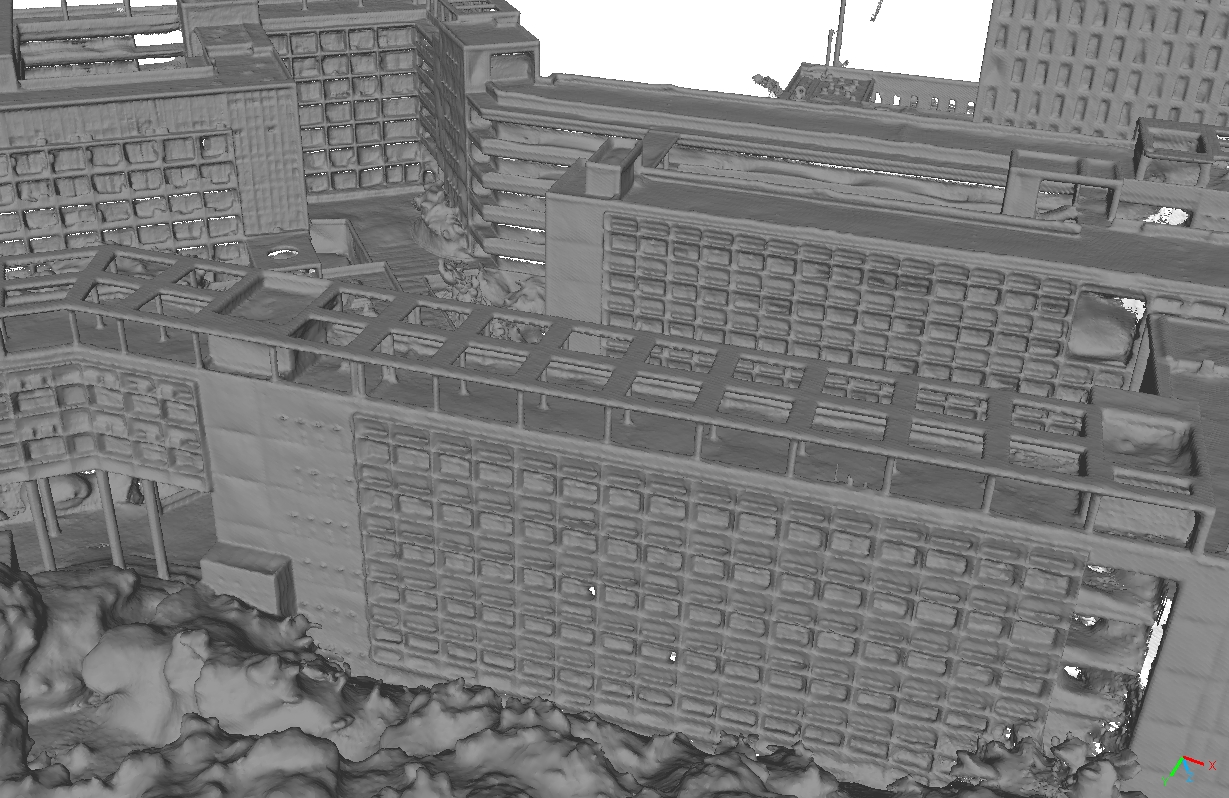
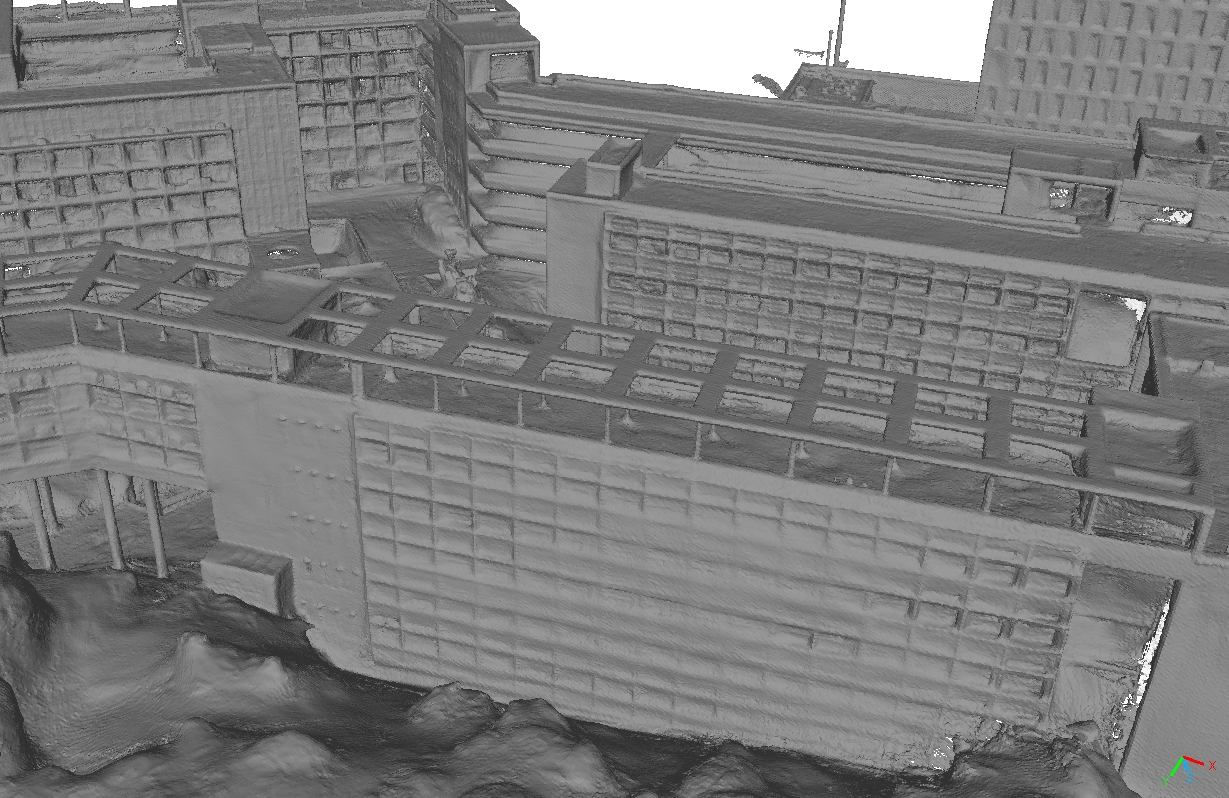
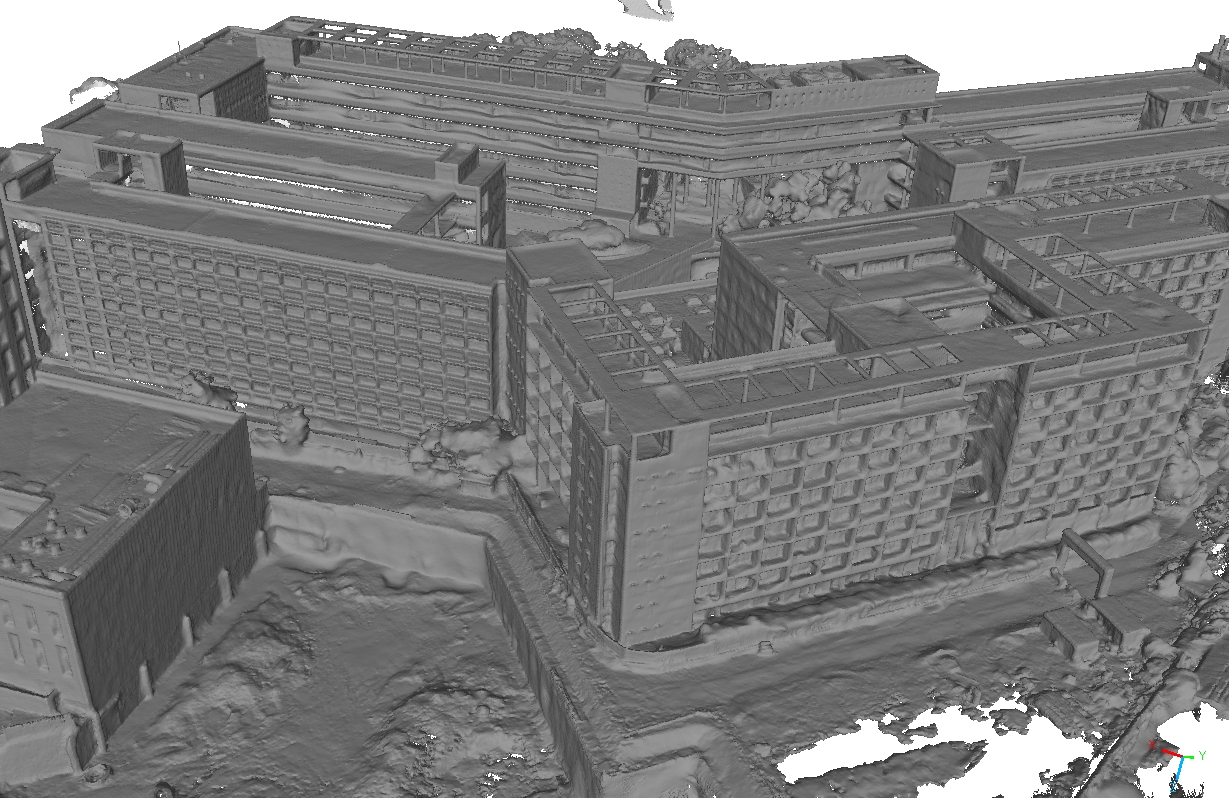
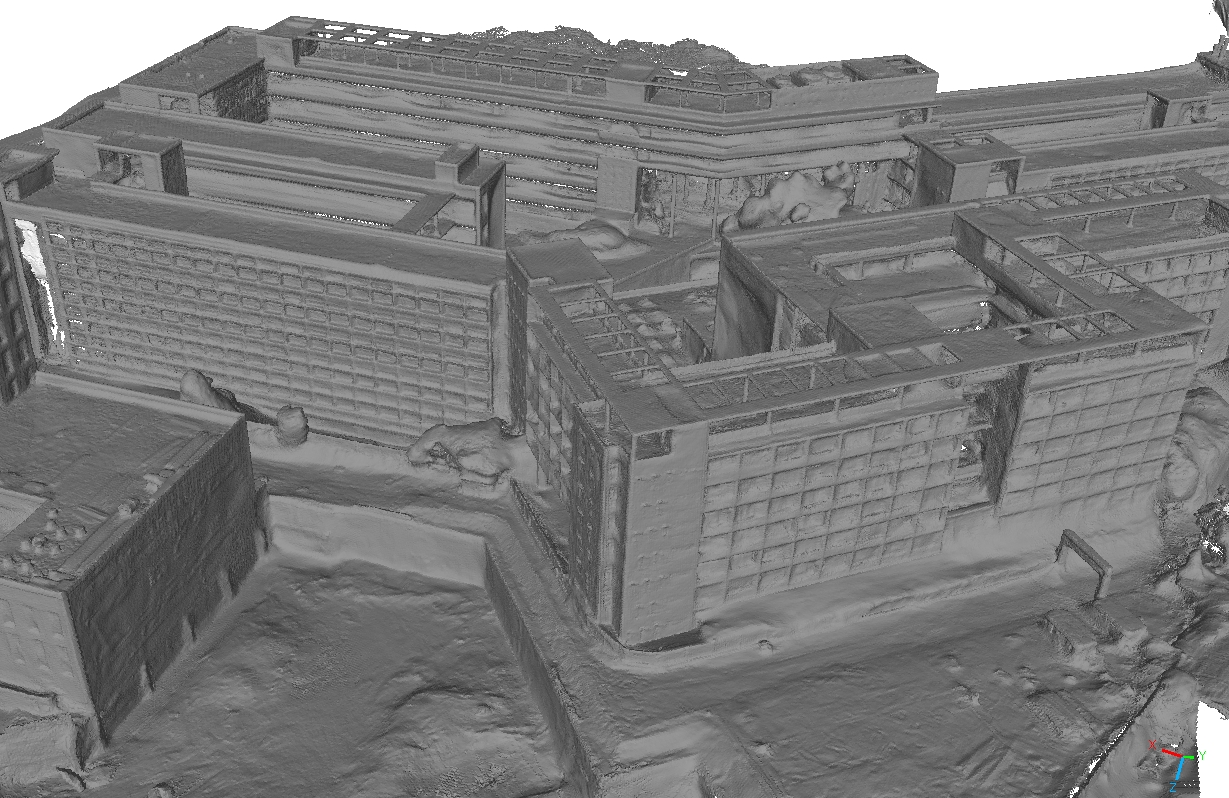
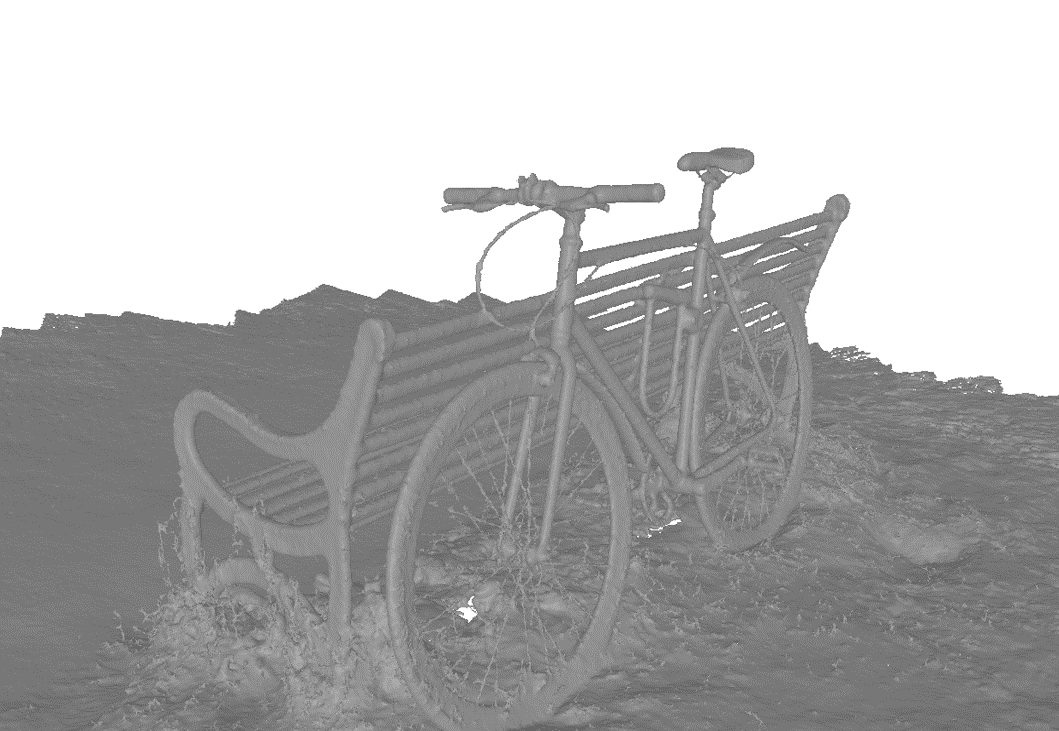
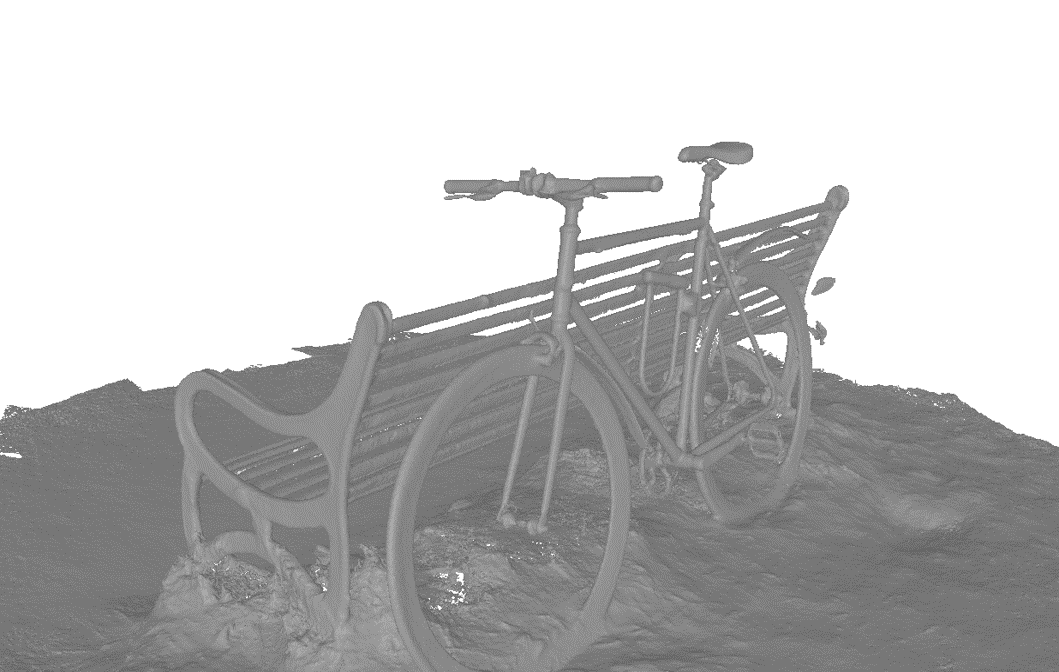
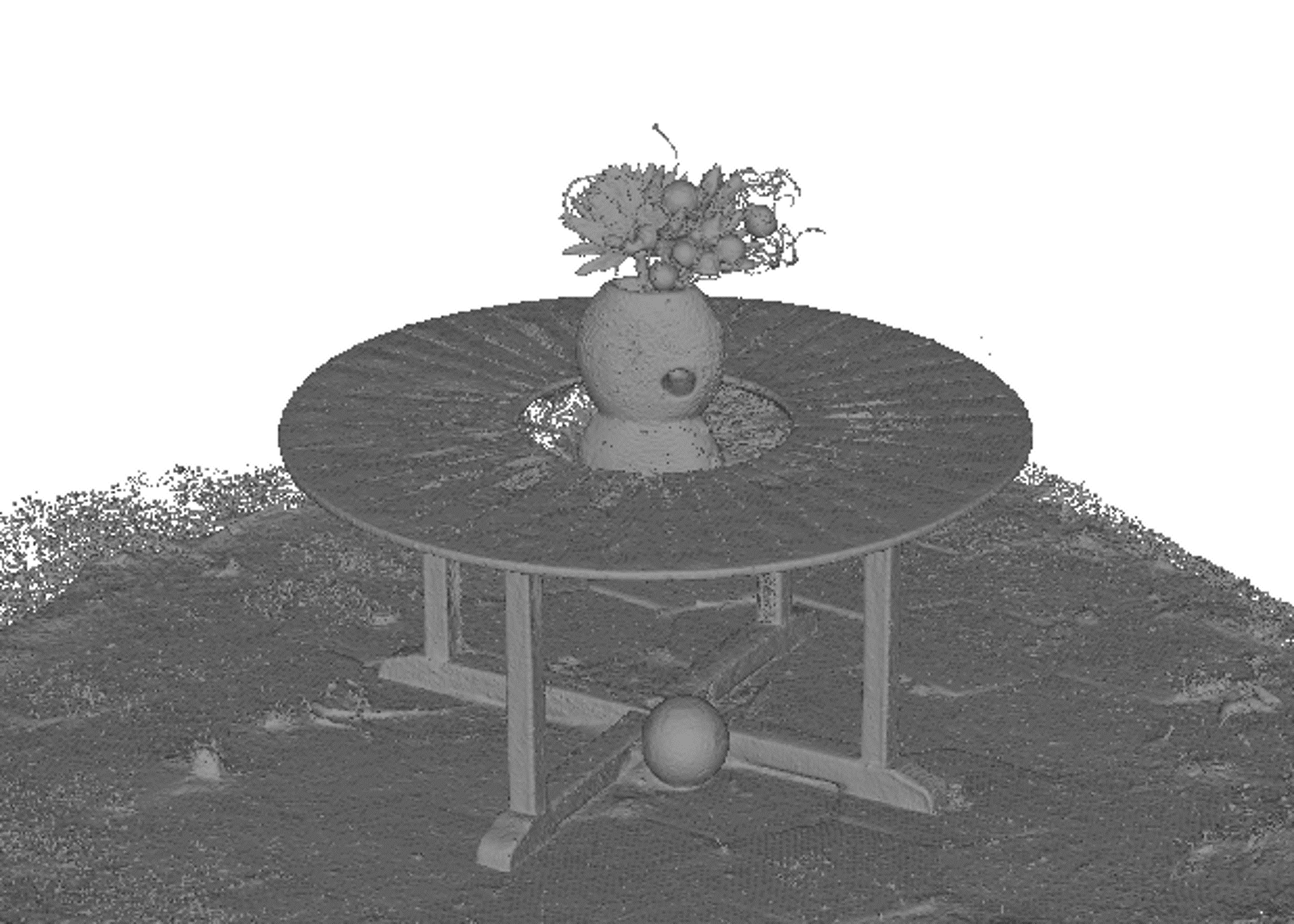
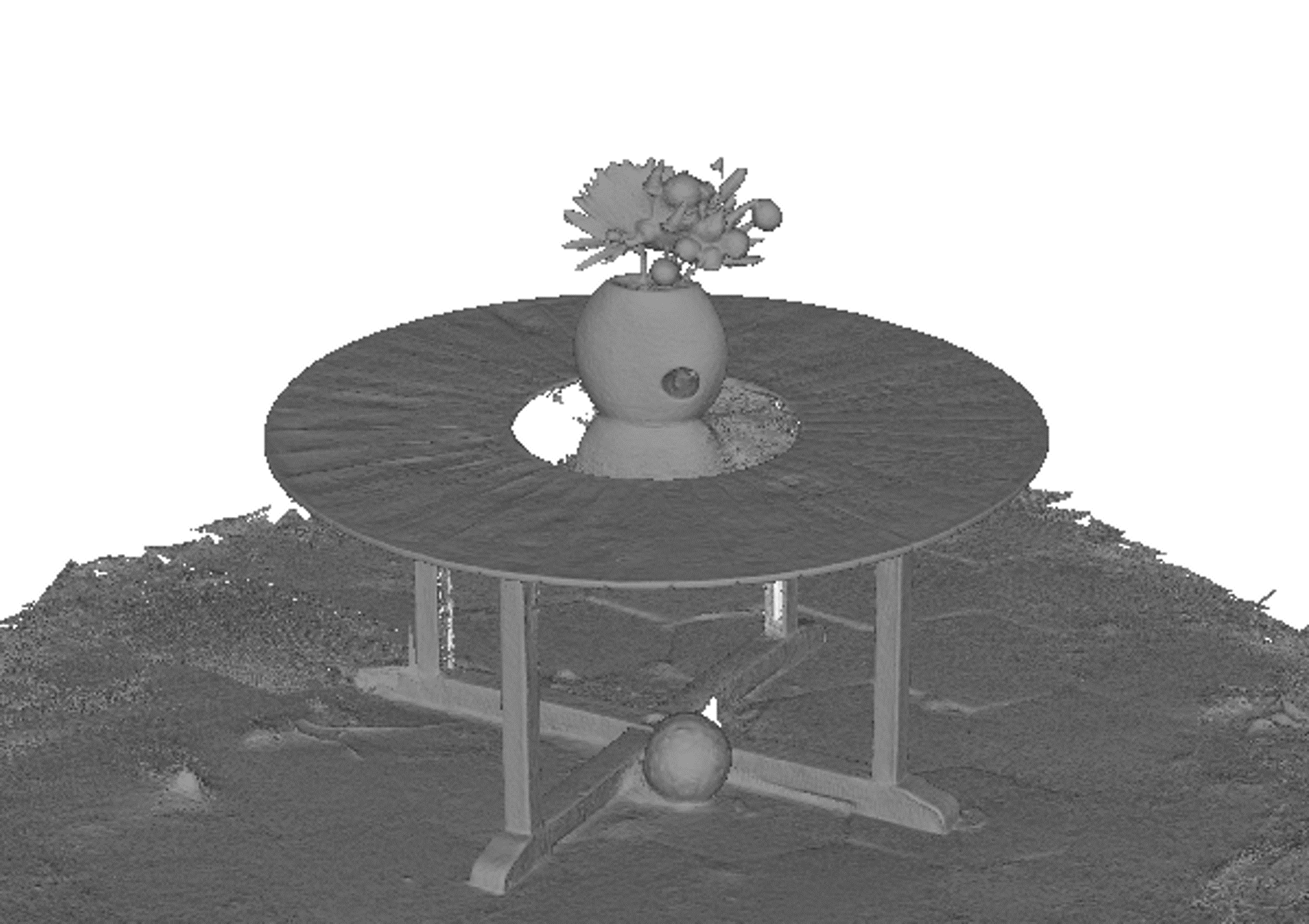
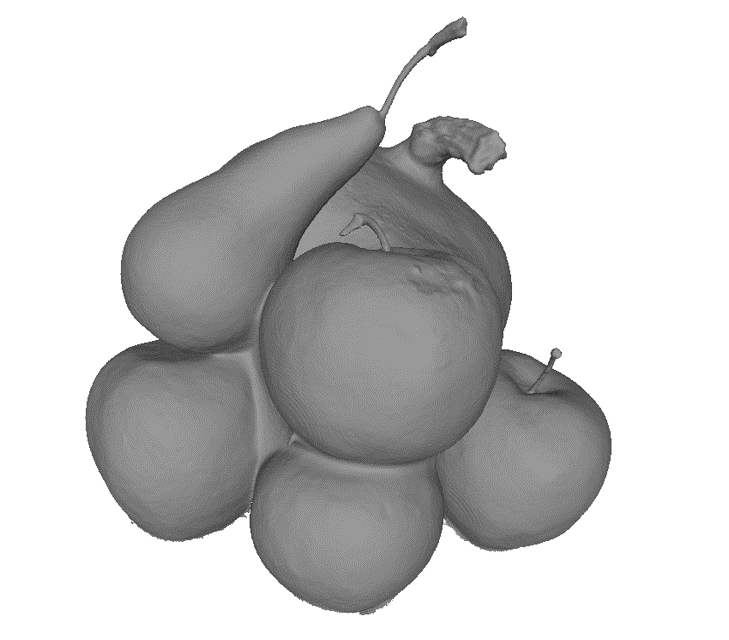
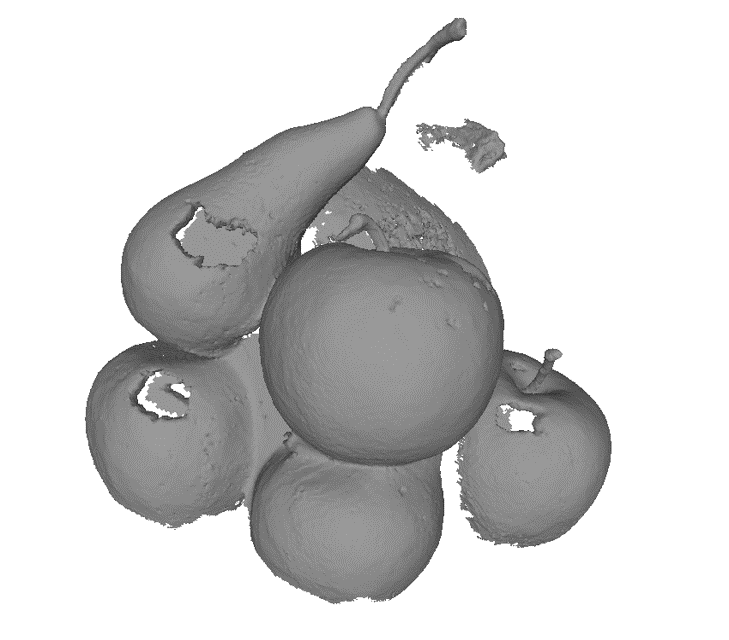
Citation
If you want to cite our work, please use:
@misc{li2024mvgsplatting, title={MVG-Splatting: Multi-View Guided Gaussian Splatting with Adaptive Quantile-Based Geometric Consistency Densification}, author={Zhuoxiao Li and Shanliang Yao and Yijie Chu and Angel F. Garcia-Fernandez and Yong Yue and Eng Gee Lim and Xiaohui Zhu}, year={2024}, eprint={2407.11840}, archivePrefix={arXiv}, primaryClass={cs.CV}, url={https://arxiv.org/abs/2407.11840}, }
Acknowledgements
The website template was borrowed from Michaƫl Gharbi and MipNeRF360.